Using Artificial Intelligence for More Personalized Ovarian Cancer Treatment
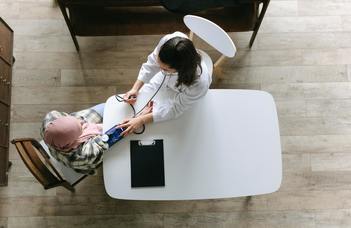
A team of researchers from Department of Physics of Complex Systems at Eötvös Loránd University, Harvard Medical School, the Danish Cancer Institute, and Semmelweis University has developed an innovative approach to predict how patients with High-Grade Serous Ovarian Cancer (HGSOC) respond to chemotherapy. Their findings, to be published in NPJ Precision Oncology, a journal of the Nature group, with Oz Kilim, an ELTE PhD student, as the lead author, demonstrate that combining two key sources of information—images of tissue samples (histopathology) and detailed protein measurements (proteomics)—leads to improved accuracy in identifying which patients are likely to benefit from platinum-based chemotherapy, a common first-line treatment for ovarian cancer.
The study introduces an AI method that pairs detailed tissue images with molecular-level data to uncover patterns that doctors might not otherwise see. This approach significantly improves predictions compared to using either type of information on its own, providing a more comprehensive picture of how a tumor behaves.
Seeing Both the Forest and the Trees
Typically, doctors rely on detailed tissue slides (H&E-stained images) to diagnose cancers and assess their type, while proteomics offers insight into the proteins within a tumor, which can indicate its vulnerabilities or resistance to treatment. Imagine trying to predict how a plant will grow using only its appearance or only the quality of the soil—it’s useful but incomplete. By combining both perspectives, the researchers’ approach is akin to studying both the plant and the environment it grows in, providing a fuller understanding.
The study tested this method on two large datasets from international cancer research centers. Results showed that combining tissue and protein data led to predictions that were significantly more accurate than existing tools, such as those based on genetic testing. This means that doctors may soon have access to more reliable tools to decide whether platinum-based chemotherapy is the best option for a patient or whether alternative treatments should be considered earlier.
A Step Toward Personalized Cancer Care
One of the most important aspects of this research is its potential to help tailor treatment plans for patients. By identifying which patients are unlikely to respond to platinum-based chemotherapy, doctors can reduce the risk of unnecessary side effects and explore other therapies sooner. Additionally, the models used in this study can also highlight why certain treatments work or don’t work, offering valuable clues for future research.
The researchers also found that the regions around tumors (the stroma) may hold important information about treatment response, which has often been overlooked. This highlights the importance of studying not just the tumor itself but also its surroundings.
Looking Ahead
While more research is needed to validate these findings across larger groups of patients, this study demonstrates the promise of combining multiple sources of data to improve cancer treatment. Future efforts could expand this approach to include other data types, such as genetic or imaging data, to further refine predictions and ensure more personalized care.
This work, to be featured in NPJ Precision Oncology, represents a collaborative effort from leading institutions in cancer research. It is an encouraging step toward using technology to help doctors make more informed decisions, improving outcomes for patients with ovarian cancer.
Original Publication:
Kilim, O., Olar, A., Biricz, A., Madaras, L., Pollner, P., Szállási, Z., Sztupinszki, Z., and Csabai, I., 2025. Histopathology and proteomics are synergistic for high-grade serous ovarian cancer platinum response prediction. npj Precision Oncology, 9(1), p.27. Link: https://www.nature.com/articles/s41698-025-00808-w
(Cover image: pexels.com)